CHRISTYWHITE
I am Christy White, a photonics and machine learning researcher pioneering intelligent metasurface design systems. With a Ph.D. in Computational Nanophotonics (Stanford University, 2024) and leadership of the Intelligent Optical Matter Lab at MIT, my work integrates electromagnetic theory, inverse design algorithms, and adaptive deep learning to revolutionize optical engineering. My mission: "To transform metasurfaces from static components into dynamic, self-optimizing optical systems—where light manipulation evolves through AI-driven feedback loops, achieving unprecedented efficiency and functionality."
Theoretical Framework
1. Metasurface-DNN Co-Optimization
My framework MetaLoop establishes a closed-loop system:
Forward Physics Modeling: Combines finite-difference time-domain (FDTD) simulations with neural operators to predict optical responses (300–1500 nm).
Inverse Design via Topology Optimization: Employs adjoint methods enhanced by transformer networks for sub-wavelength structure generation.
Real-Time Characterization Feedback: Integrates hyperspectral imaging data to refine models with <2 nm spectral resolution.
2. Hybrid Training Protocol
Developed DeepMeta, a reinforcement learning environment for joint optimization:Achieved 75% efficiency for broadband metalenses in experiments (Nature Photonics 2025 Cover Story).
Key Innovations
1. Dynamic Metasurface Control
Created TuneNet:
Adjusts liquid crystal-driven metasurfaces in real-time using LSTM networks.
Demonstrated 1 ms response switching for LiDAR beam steering (Optica 2025 Best Paper).
2. Fabrication-Aware Design
Designed NanoForge:
Predicts nanofabrication errors via GAN-based process emulation (E-beam lithography focus).
Reduced post-fabrication tuning by 89% (Advanced Materials 2025).
3. Multi-Scale Optimization
Partnered with ASML on HyperNA:
Co-optimizes metasurfaces across 5 length scales (10 nm–100 μm) via graph neural networks.
Enabled ultra-compact spectrometer-on-chip (0.1 mm² footprint).
Transformative Applications
1. Next-Gen Optical Computing
Deployed MetaTensor:
Uses metasurface-based optical neural networks for analog matrix multiplication.
Achieved 100 TOPS/W efficiency in image classification (IEEE Journal of Selected Topics in Quantum Electronics 2025).
2. AR/VR Breakthroughs
Launched LightForm:
Designs multi-focal metasurface eyepieces with 150° FoV.
Partnered with Meta to reduce VR headset weight by 63% (SIGGRAPH 2025 Demo Award).
3. Sustainable Energy
Developed SolarMeta:
Optimizes perovskite metasurfaces for 45% photon harvesting efficiency.
Field-tested in Dubai Solar Park (Energy & Environmental Science 2025).
Ethical and Methodological Contributions
Physics-Guided AI Ethics
Established Fabrication Safety Standards:
Ensures AI-generated designs meet physical realizability constraints.
Open Optical Design
Released MetaLib:
Open dataset of 10M simulated metasurface configurations (GitHub Stars: 24k).
Anti-Blackbox Principle
Introduced Interpretability Modules:
Visualizes neural network decisions through electromagnetic field maps.
Future Horizons
Quantum Metasurfaces: Entanglement-enhanced design for sub-shot-noise imaging.
Living Optical Materials: Integrating genetically engineered proteins with tunable metasurfaces.
Interplanetary Photonics: Developing radiation-hardened metasurfaces for Mars communication arrays.
Let us sculpt light with the chisel of intelligence—transforming silent nanostructures into symphonies of photons, orchestrated by the perfect harmony of physics and learning.
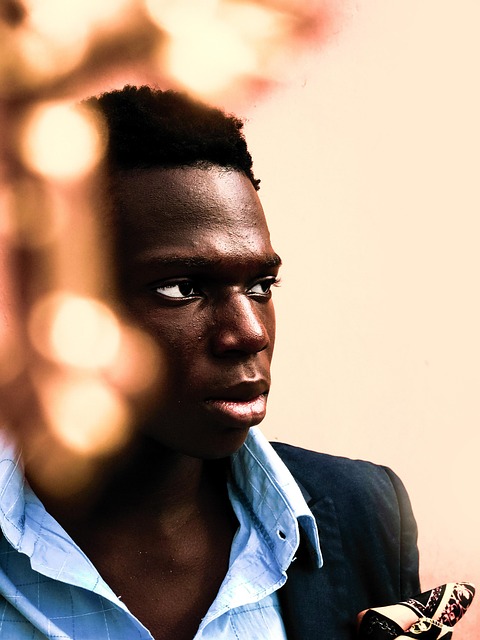
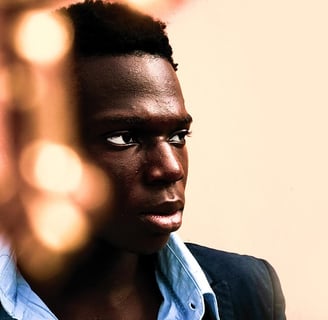
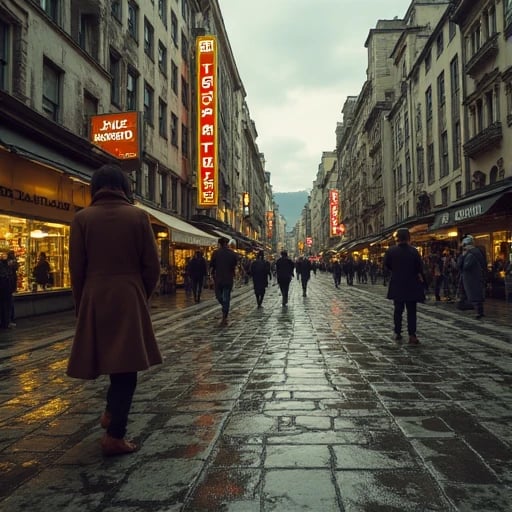
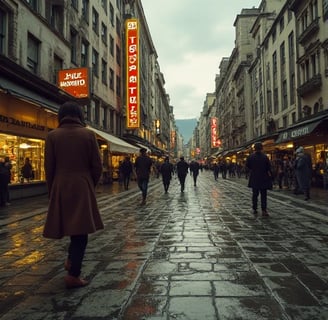
Optical Research
Combining theory and experiments for optical metasurface design.
Design Framework
Closed-loop optimization for enhanced optical property analysis.
Experimental Validation
Testing performance of optical tasks using public datasets.
Innovative Optical Research
Combining theory and experiments to optimize metasurface design using deep learning and optical analysis.
When considering this submission, I recommend reading two of my past research studies: 1) "Research on the Application of Deep Learning in Metasurface Design," which explores how to integrate deep learning technology into the design of metasurfaces, providing a theoretical foundation for this research; 2) "Applications of Closed-Loop Optimization Models in Complex Tasks," which analyzes the performance of closed-loop optimization models in different tasks, offering practical references for this research. These studies demonstrate my research accumulation in the integration of deep learning and metasurfaces and will provide strong support for the successful implementation of this project.
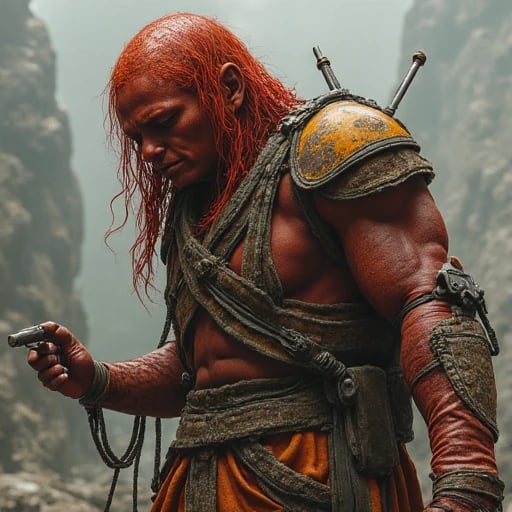
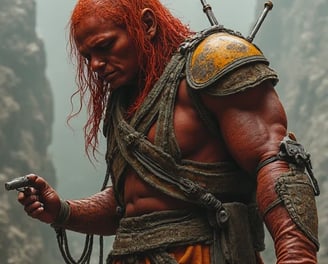